Building Large Models at a Fraction of the Cost: FLM-101B Strategy
Written on
Chapter 1: The Financial Landscape of AI
Today, we delve into a significant topic in the AI realm—finances. Contrary to what you might think, this discussion is far from dull. In fact, the influence of financial considerations on AI is profound, shaping both technical and strategic decisions across the industry.
In a recent announcement, a group of researchers revealed an extraordinary achievement that could change the game.
This piece was first published in my weekly newsletter, TheTechOasis. If you're interested in staying informed about the fast-paced world of AI and looking for inspiration to navigate the future, consider subscribing below:
TheTechOasis
The newsletter to stay ahead of the curve in AI
thetechoasis.beehiiv.com
The Underlying Cost Dilemma
"Costs are a major concern," is likely what Sam Altman, OpenAI's CEO, contemplates regularly. Analytics India Magazine suggests that OpenAI might face bankruptcy by 2024. However, I find it hard to believe that scenario will unfold, given that OpenAI is on track for $1 billion in revenue. Nevertheless, the daily operational costs of running models—approximately $700,000—illustrate the financial challenges present in AI.
Whether it involves training or model inference, the expenses are substantial. Using Microsoft Azure as a benchmark, operating an 8-GPU NVIDIA A100 cluster on their platform incurs costs of about $27 per hour. Given that training large models can take months, costs can escalate rapidly. For context, the initial LLaMa models required $5 million for just 21 days of training their 65-billion-parameter model. With GPT-4 being a trillion-parameter model, Sam Altman's estimate of around $100 million in total costs is not far-fetched.
As the correlation between model size and quality continues to hold true, the race to create larger models intensifies, often at significant financial expense. Yet, researchers argue that this need not be the case.
Identifying Current AI Challenges
Researchers have identified several issues in existing AI training methodologies:
- Cost: Training or operating models is prohibitively expensive for all but a select few corporations and governments.
- Extrapolation Limitations: Current LLMs often underperform when processing longer text sequences than they were trained on, leading companies like OpenAI to restrict such capabilities.
- Evaluation Standards: The methods used to assess LLMs do not accurately reflect their intelligence, as evaluations are based solely on their knowledge and task performance.
The researchers' goals are clear:
- Demonstrate that large models can be built at dramatically lower costs.
- Develop a model that handles longer sequences effectively.
- Create a new evaluation standard that better measures a model's intelligence.
If successful, this research could significantly impact AI development.
The Path to Cost-Effective Model Building
As we discussed previously, the appeal of open-source software is compelling, yet often out of reach. To address training costs, researchers propose a bold growth strategy. Instead of constructing three distinct models from scratch, as done by firms like Meta or OpenAI, the paper introduces FLM-101B—a 101-billion-parameter model developed through a phased approach:
- Start with a 16-billion parameter model.
- Build a 51-billion model based on the initial 16-billion model.
- Finally, create the 101-billion model from the previous two.
This strategy significantly shortens the time required to develop a high-performance model from 48 GPU days to just 22 days.
How substantial are the cost savings? By adopting this method, the overall GPU training days decrease, along with the amount of training data needed for each model iteration. Similar-sized models typically require a dataset of around 300 billion words for initial training, but FLM-101B utilizes a scaled approach, as illustrated below:
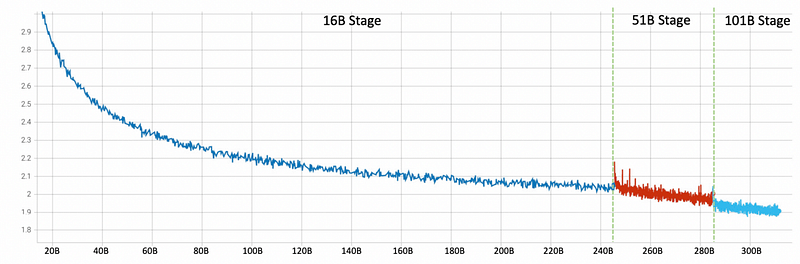
As depicted in the image, most training data is allocated to the smaller 16B model, with larger models extending from this foundation while requiring significantly less data. In essence, the training time using 300 billion tokens is reduced, resulting in a dramatic decrease in the number of floating point operations (FLOPs), which measure GPU usage and associated costs.
But why is this effective? Recent findings have confirmed the concept of function preservation, indicating that the knowledge a smaller model acquires can be leveraged by larger models during their training. This leads to the conclusion that while training a 101B model from scratch might cost roughly $10 million, FLM-101B can be built for just $100,000—representing a 100-fold reduction in expenses.
This makes the creation of large models financially feasible.
Addressing Extrapolation through xPos
Regarding extrapolation, the researchers employed xPos positional embeddings. These embeddings are essential for transformers like ChatGPT, as they provide critical order information about token sequences. By utilizing rotary positional embeddings (RoPE) and the xPos method, they ensure that positional embeddings do not hinder model performance during extrapolation.
Most transformer models experience significant performance drops with longer sequences than anticipated. To mitigate this, companies like OpenAI impose restrictions. Extrapolation is vital for achieving AGI, as machines should emulate human-like abilities to extrapolate.
The researchers also proposed a novel methodology for evaluating LLM intelligence, addressing a crucial question: How do we determine if a model is reasoning or merely recalling information?
One proposed evaluation method, "anti-interference," challenges the model to perform well in noisy scenarios, compelling it to discern relevant data from irrelevant information.
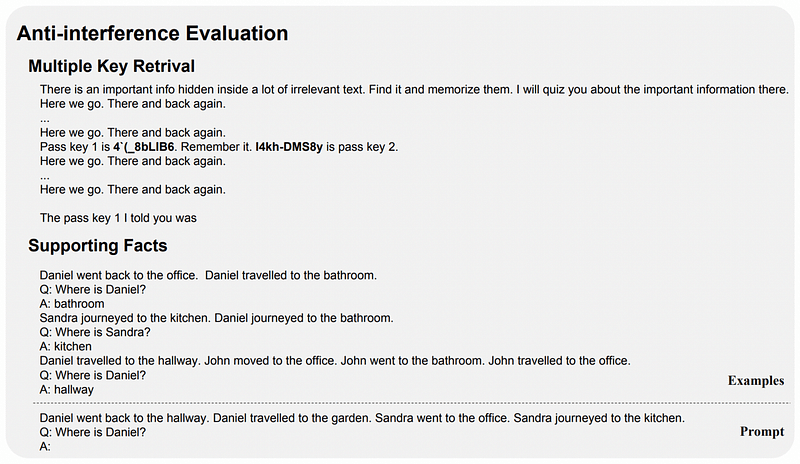
A Breath of Fresh Air for the Industry
While many may not directly utilize FLM-101B, its development serves a broader purpose: to elevate the AI industry by introducing a viable training method and shedding light on new insights regarding LLM intelligence evaluation.
Moreover, it's worth noting that this research originates from China, highlighting a positive trend of openness in the global AI community.
Link to research paper
Subscribe to DDIntel Here.
DDIntel highlights notable pieces from our main site and popular DDI Medium publication. Check us out for further insightful contributions from our community.
Register on AItoolverse (alpha) to earn 50 DDINs.
Follow us on LinkedIn, Twitter, YouTube, and Facebook.
Chapter 2: Innovative Approaches in AI Development
In exploring new methodologies, researchers are embracing innovative strategies to reshape the AI landscape.
The first video discusses the differences in embedding costs between OpenAI and alternative open-source options, providing context to the financial aspects we’ve explored.
The second video reveals the most effective alternatives to ChatGPT, showcasing how leveraging various LLM models can empower users.